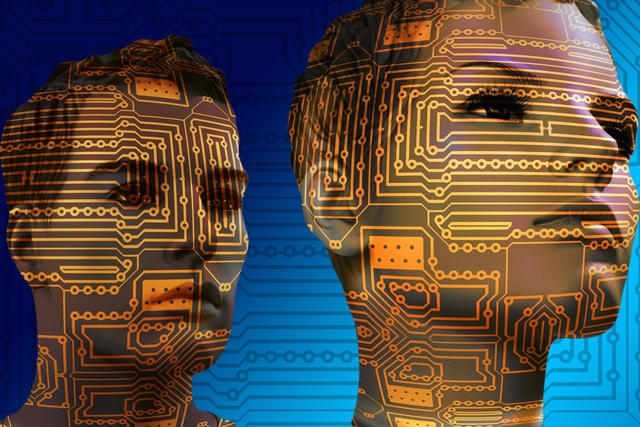
(Gerd Altmann, Pixabay)
12 Dec. 2018. The use of artificial intelligence continues to grow both in new applications and complexity of the problems they address. A law school professor urges developers, users, and regulators of machine learning in medicine to validate the algorithms that represent their underlying decision-making processes, in today’s issue of the journal Science Translational Medicine (paid subscription required).
The need for more transparency in medical machine learning algorithms is outlined by W. Nicholson Price, a professor of law at University of Michigan, as well as at University of Copenhagen in Denmark. Price describes the expanding use of machine learning algorithms in decision-support software in radiology, pathology, and diagnostics. He cites tests of algorithms showing they interpret images of skin lesions as well as board-certified dermatologists, and can identify trauma patients for risk of hemorrhage without constant expert consultation. In addition, algorithms are filtering down to smartphone apps to identify, for example, developmental disorders in children, and expanding to decisions on allocating resources in health systems.
One problem Price notes is the opaque nature of these machine-learning routines, which he calls black box algorithms. While these algorithms can provide interpretations, recommendations, and predictions, they typically do not provide an explanation of how they compute those interpretations, recommendations, and predictions. And as a result, a tension emerges between restricting algorithms to more mechanistic and easily understood models, or trusting results of block-box algorithms on highly complex and nuanced problems without question.
Also, machine-learning algorithms are almost by definition constantly changing, a feature Price calls plasticity. As these algorithms encounter new data, they’re designed to adjust their computations to reflect the richness and complexity of those data. In contrast, medical interventions are largely designed to remain stable, with consistent quality a prized feature of drugs and medical devices.
3-step validation process
These features of opacity and plasticity, says Price, make it imperative that medical A.I. algorithms are reviewed and rigorously evaluated. He proposes a 3-stage process that applies consistent standards, but allows as well for flexibility and adjustments as algorithms encounter new data. The first stage is procedural, where the algorithm’s underlying model is reviewed for real-world accuracy and the data for initially training the algorithm are vetted for quality.
The second validation stage is reliability testing, where algorithms are assessed against independent data sets, and not only against the data they encounter from current and previous users. And the third validation step is performance reports showing the extent of success or failure in dealing with real-world cases. Price notes that these performance reports can also serve as training data for new algorithms and for updating existing routines with hard day-to-day evidence.
In the U.S., the Food and Drug Administration plays a special role, since the agency regulates medical devices. Price cites recent draft guidance and planning documents issued since December 2017 showing FDA recognizes that role with what the agency calls clinical decision support software. However, FDA’s enabling legislation requires an initial risk-based analysis that can exempt many machine learning algorithms, and also calls for a one-time authorization of medical devices, while black box algorithms are by definition constantly changing as they engage new data.
A key issue noted by Price is the need for better tools for regulatory oversight of black-box algorithms, with conventional clinical trials largely inadequate for the task. As described in Science & Enterprise in September, a new type of adaptive clinical trial that adjusts with the evidence it encounters, much like machine-learning algorithms, is gaining traction. One such trial is testing breast cancer treatments based on patients’ biomarkers and MRI images, and employs an adaptive design with algorithms that make it possible to alter factors such as treatment regimens or sample sizes based on interim results.
In addition, FDA earlier this month recognized a public database of genomics and diseases to provide independent data sets for assessing diagnostics tests using genetic data for precision medicine. This kind of database could also offer data for independent evaluations of black box algorithms.
More from Science & Enterprise:
- Project to Catalog Precision Lung Cancer Immunotherapies
- Personalized Computer Models Developed for Heart Disease
- NVIDIA, Scripps Partner on Medical Deep Learning Applications
- Algorithms Advance Assistive Control for Spinal Cord Injury
- A.I. to Identify Treatment Drivers for Colorectal Cancer
* * *
You must be logged in to post a comment.